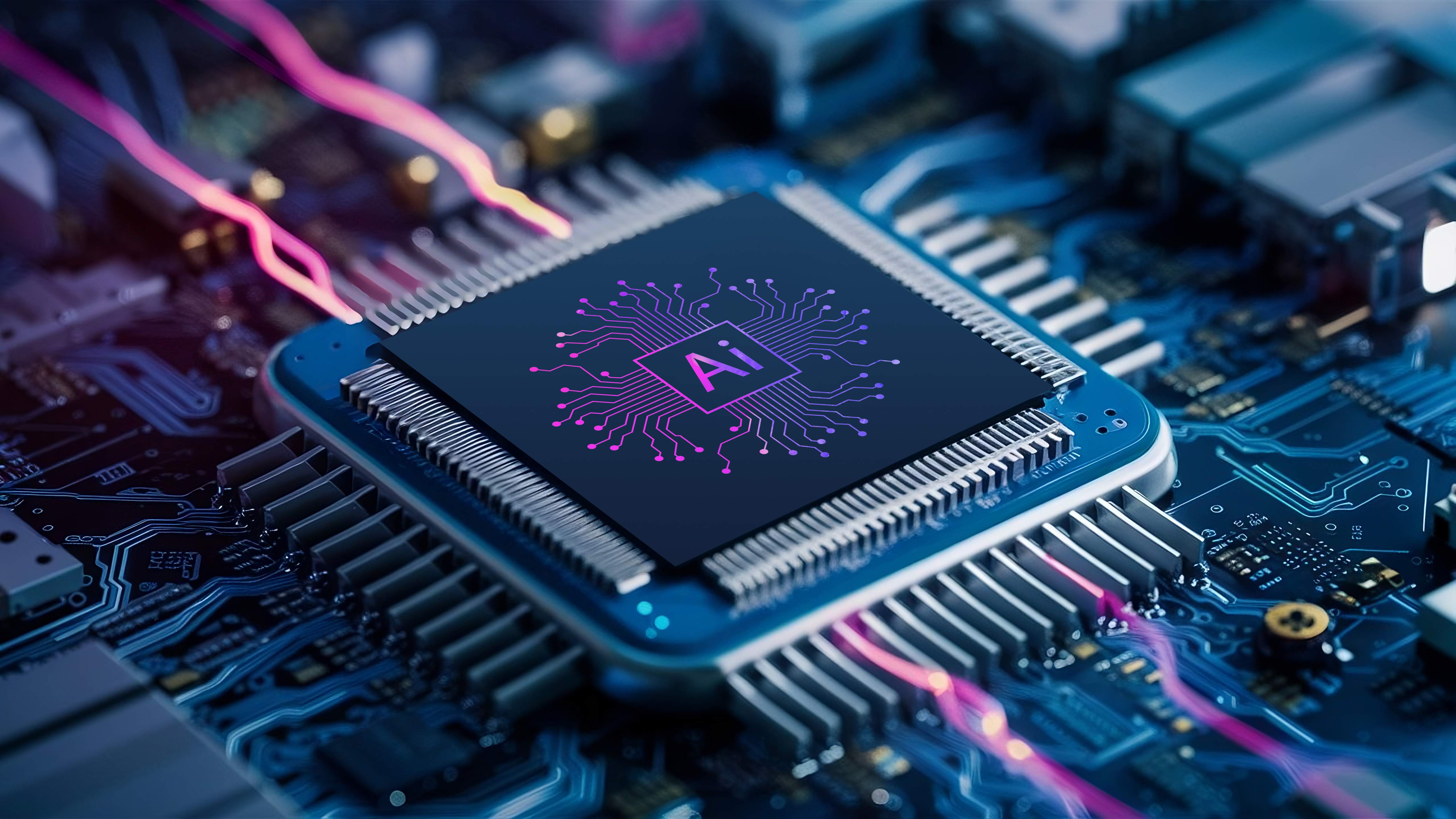
RRAM Breakthrough: BM LABS Sets the Stage for Next-Gen AI Compute-in-Memory
Jain, S., Li, S., Zheng, H. et al. Heterogeneous integration of 2D memristor arrays and silicon selectors for compute-in-memory hardware in convolutional neural networks. Nat Commun 16, 2719 (2025). Click
Singapore, February 2025 – A groundbreaking study from BM LABS team and researchers at the National University of Singapore (NUS) has demonstrated that RRAM can operate at sub-0.8V and exceed switching speeds of 1GHz, positioning it as a viable complement to SRAM in compute-in-memory (CIM) applications. Compared to Intel’s 18A SRAM at 5.6GHz and 1.05V, RRAM’s lower voltage, CIM capability, and CIM capability make it a compelling option for next-gen AI accelerators. This breakthrough marks a significant advancement in AI computing, enabling high-density, energy-efficient inference solutions that challenge traditional SRAM-based architectures.
As AI computing demands increase, traditional memory technologies face significant limitations. SRAM, while fast and widely used, struggles with power efficiency, density scaling, and cost at advanced nodes. The industry is actively seeking a non-volatile, energy-efficient complement that can deliver high-speed CIM capabilities without compromising scalability.
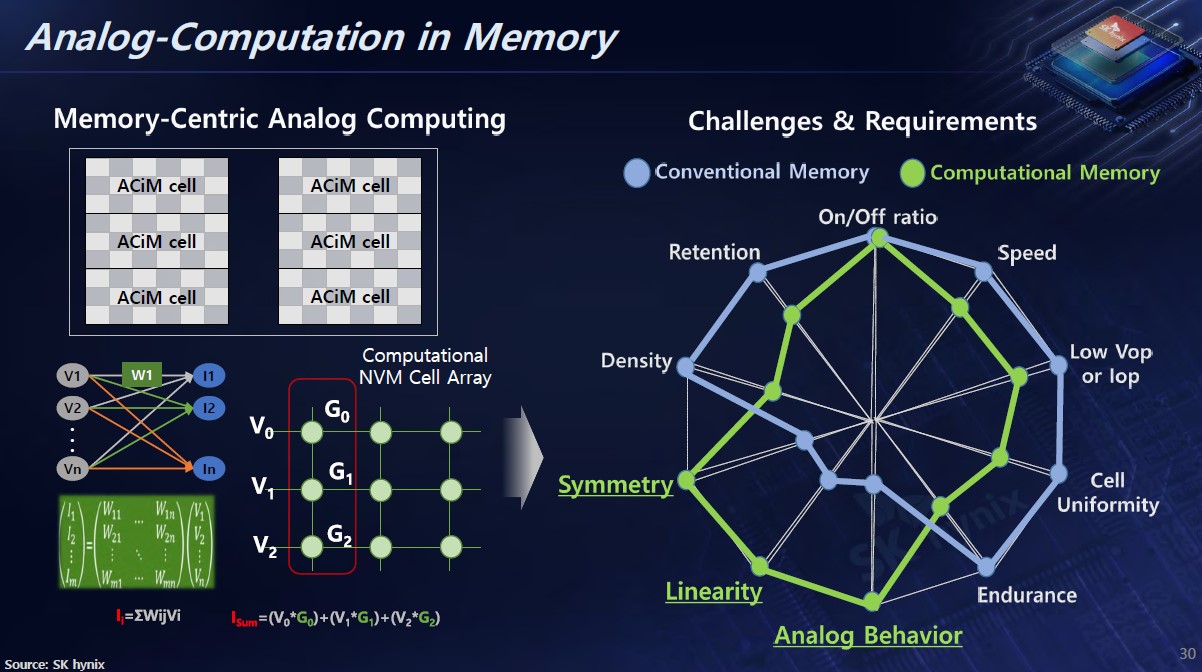
The Rise of Neuromorphic Computing
Neuromorphic computing is gaining traction as a low-power, event-driven AI processing approach, recently highlighted by Mercedes-Benz for automotive applications (source). Unlike traditional accelerators, neuromorphic chips integrate in-memory computing, making RRAM an ideal candidate for next-gen AI hardware. BM LABS is developing neuromorphic solutions to enhance pattern recognition, sensor fusion, and autonomous driving, enabling adaptive AI on edge devices without cloud dependency.
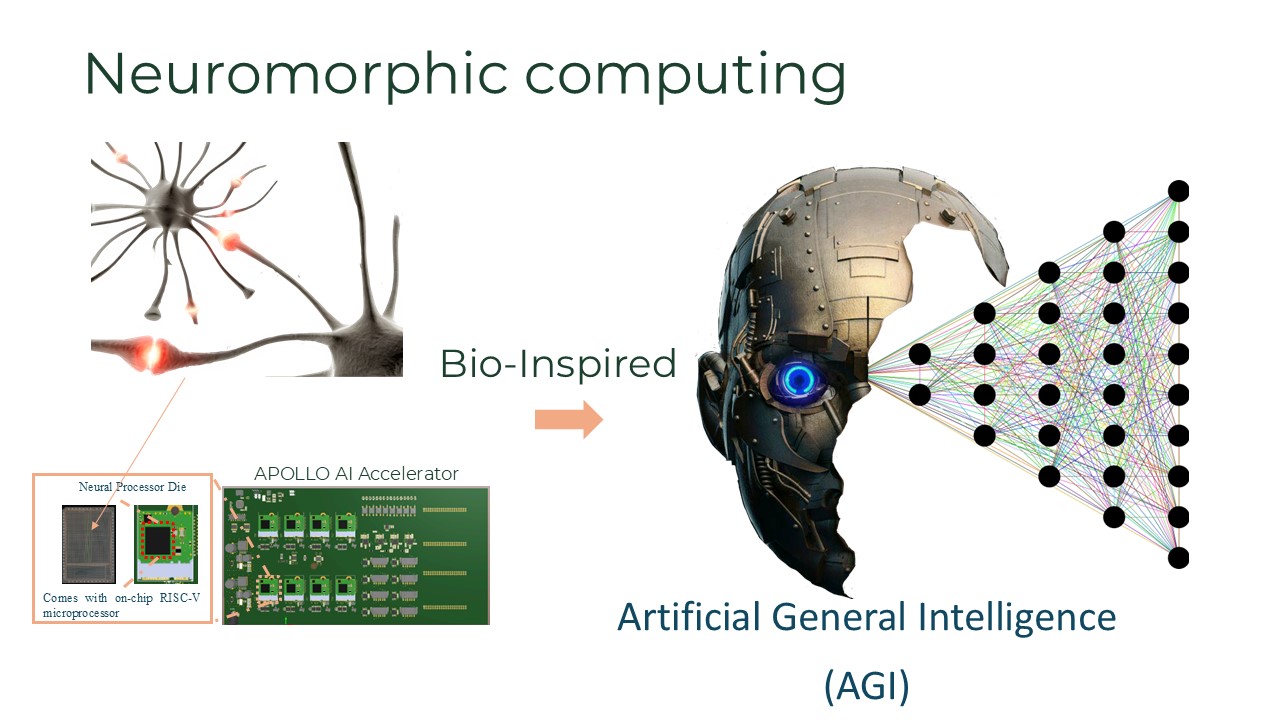
A Confirmed Roadmap for RRAM Scaling
Industry players, including Weebit Nano, have already highlighted that RRAM can scale up to 28nm and below (source), reinforcing the technology’s ability to replace eFlash in embedded applications. Moreover, RRAM is already being used in commercial products by companies like Infineon (source), Onsemi has announced plans to integrate RRAM into their future products (source), and GlobalFoundries has shown interest in RRAM (source). Additionally, SK Hynix has shown interest in RRAM (source). This confirms that existing semiconductor infrastructure can be leveraged to position RRAM for compute applications, making its transition to CIM and neuromorphic AI a natural progression.
Our research showcases a 1-kilobit (32 × 32) 3-bit RRAM cell built on a silicon-compatible 2D material platform, reducing parasitics and enabling high-speed, energy-efficient operation. This breakthrough aligns with industry efforts to move beyond traditional memory limitations and unlock the full potential of CIM and neuromorphic computing.
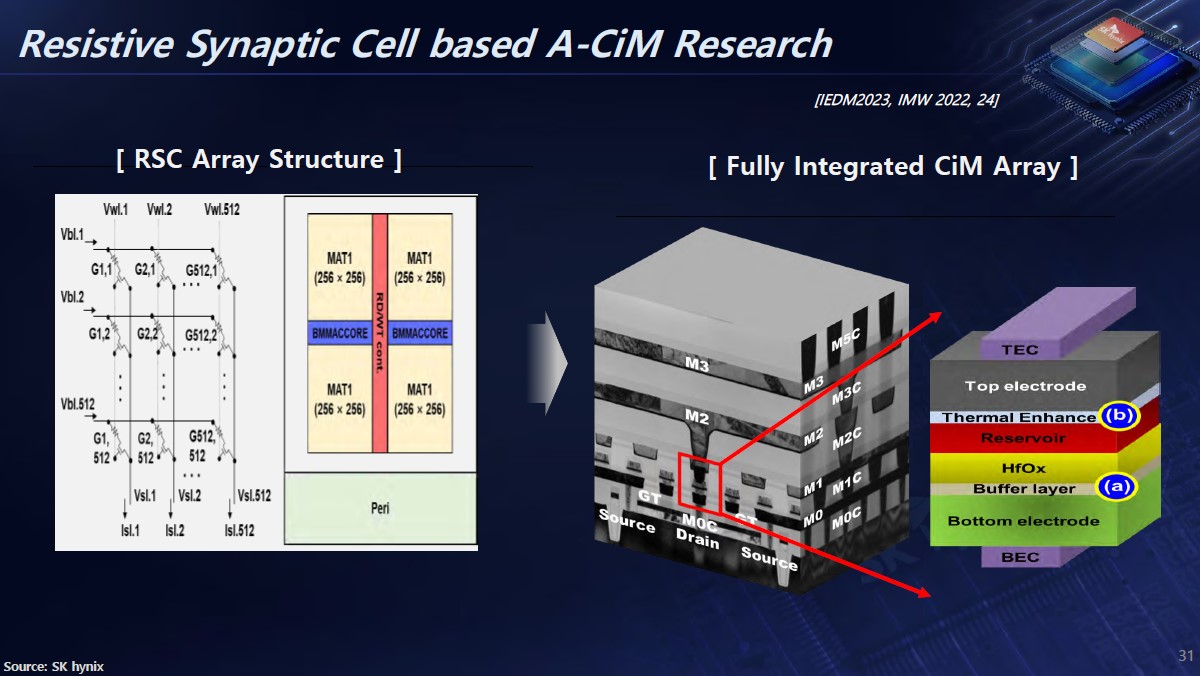
RRAM vs. SRAM: Shifting the Paradigm in AI Compute
At ISSCC 2025, Intel’s 18A SRAM demonstrated operation at 5.6GHz at 1.05V (source), while TSMC’s N2 SRAM has also made significant advancements, showcasing improved density and power efficiency, making it a strong contender in next-generation compute applications (source), reaffirming SRAM’s dominance in high-speed compute applications. However, as memory technology evolves, SRAM struggles with power efficiency and density scaling, presenting an opportunity for RRAM to take center stage in CIM and neuromorphic AI.
Comparative analysis of various AI compute-in-memory solutions highlights that while full-CMOS architectures like NVIDIA Jetson and Google TPU remain dominant, ReRAM-based architectures show significant promise in efficiency, density, and scalability. Existing alternatives like MRAM and PCM also bring advantages (source) but face limitations such as process complexity and high programming current. Our RRAM-based CIM approach overcomes these challenges, delivering superior efficiency while maintaining manufacturability on standard silicon platforms.
RRAM’s Emerging Role in the Growing AI Inference Market
As demand for low-latency AI inference increases, the RRAM-based compute paradigm is poised to capture a massive market opportunity. AI customers are increasingly willing to perform offline training but cannot compromise on inference latency, especially for edge AI applications in automotive, military, and consumer electronics sectors such as mini PCs, AR/VR, and gaming. This has driven growing interest in RRAM-based solutions, which offer low-power, high-speed processing for real-time AI tasks.
Current industry leaders, such as NVIDIA with its Jetson devices, dominate the edge inference market. However, as RRAM technology scales and improves performance, these existing solutions will face strong competition from RRAM-based CIM architectures. As AI models continue to grow in complexity and size, the need for high-density, energy-efficient inference hardware becomes even more pressing.
BM LABS is actively working on chip solutions for large AI inference models using this breakthrough RRAM technology. Our goal is to develop a scalable, high-performance AI accelerator that competes directly with existing SRAM-based and GPU-based inference solutions, positioning RRAM as the future of ultra-low-latency AI computing.
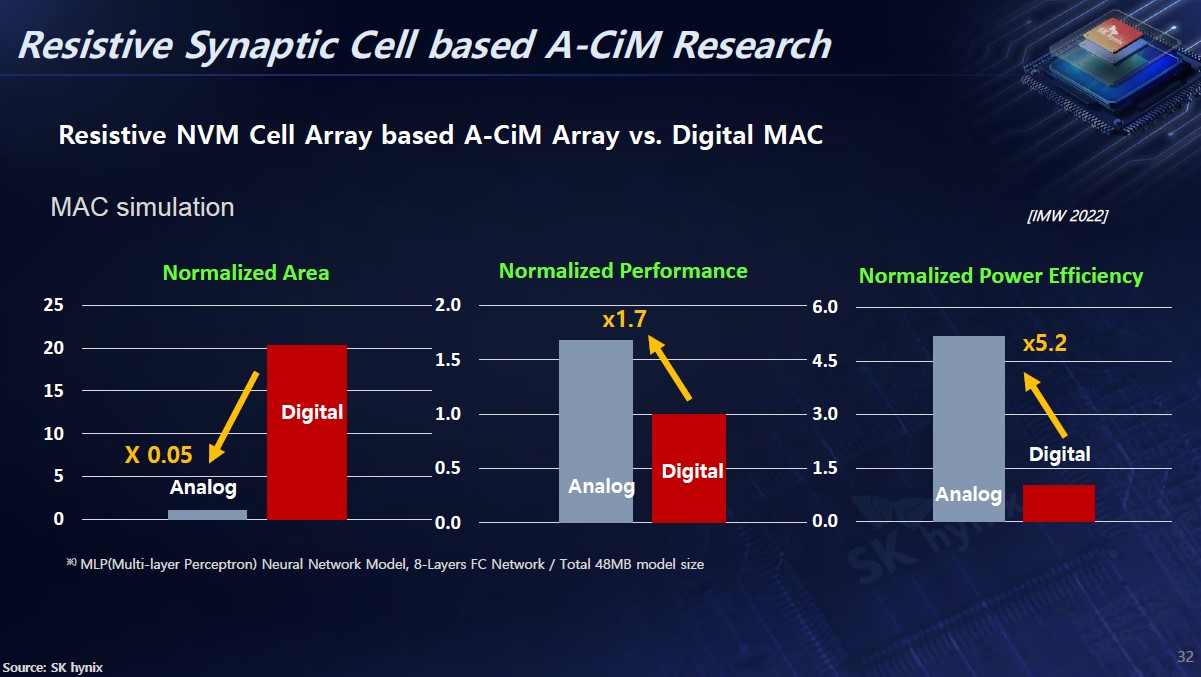
About BM LABS
BM LABS is a fabless company developing AI accelerators for fast inference using advanced memory technologies like RRAM. It is actively engaging with semiconductor companies, AI accelerator developers, and automotive AI leaders to accelerate RRAM-based CIM adoption.